If you missed our last blog, which looked at the perceived lack of representativeness in online data, you can read it here.
Next up in our blog series on AI myths, we address the common misconception that traditional quantitative methods are the sole route to meaningful insights. While obviously valuable, these approaches can overlook AI’s unique power to analyse vast datasets, revealing hidden patterns and deeper customer understanding.
Let’s explore how AI quantification benefits in ways that we could have never imagined twelve months ago.
Answers to business questions are often already out there
While generative AI excels at summarising information, it often lacks the ability to quantify it. This is where our bespoke AI solutions come in. They allow us to delve deeper into specific topics on a much larger scale than traditional quantitative research.
We believe in using a mix of methodologies and data sources to draw out the most accurate conclusions. The answers to your business questions might already exist! Our job is to find and unlock the value of that data (from surveys, images, audio, videos, documents, call transcripts, or even publicly available data like reviews, social media posts, and forum discussions).
And we leverage subscriptions, APIs and bespoke webscraping to collect our own proprietary data to help inform customer strategy. For example, we recently helped a B2B client who wanted to understand their customers’ needs and pain points better in order to reduce churn.
Traditional quantitative methods weren’t feasible because they wanted to analyse a specific subset of customers and 10 years’ worth of call center recordings. Our bespoke AI solution allowed them to rapidly extract valuable insights from this vast amount of unstructured data.
Traditional AI does quantify, and sometimes on a deeper level
A powerful tool for analysing unstructured data is topic modelling. It allows us to analyse massive amounts of customer journey data, including real-time (in the moment) and unprompted feedback.
For example, imagine analysing headphone reviews. Topic modelling can break down this information into specific themes or topics, which we can then compare with meta data like star ratings, markets, brands, and product descriptions.
In a recent project, we used web scraping to collect and analyse around 200,000 public reviews across 30+ brands in 10 markets. Topic modelling, part of Natural Language Processing (NLP), lets us take a top-down approach to understanding the data. We can identify overall strengths and weaknesses before diving into the verbatims and distinctive wording of the reviews to help inform brand positioning.
Here, AI isn’t being used to replace brand tracking, but to compliment it. Online data is no substitute for traditional brand funnels (awareness, familiarity, consideration etc). But for smaller brands it can be incredibly valuable – obtaining a large volume of real customer reviews can be a challenge.
In our example, topic modelling allowed us to analyse thousands of reviews from actual product owners, compared to the potentially limited 30 people who might have participated in a traditional quantitative brand tracker survey. Using both sets of data together provides a much richer picture.
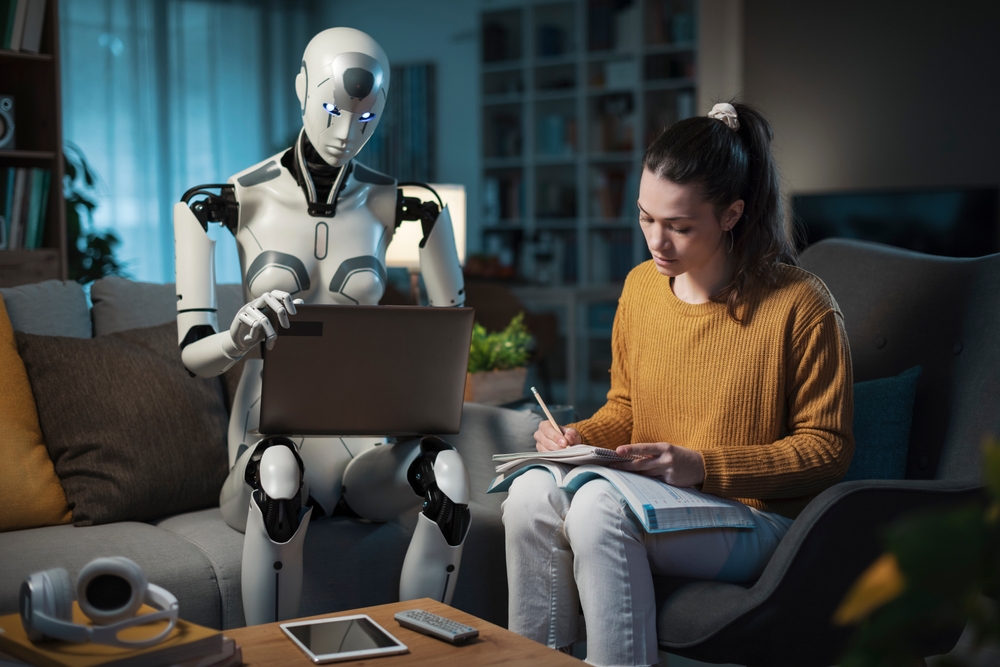
Reveal fresh perspectives
It’s also worth going beyond quantitative and online data to uncover hidden insights. When a direct conversation with customers seems most valuable, we have the tools to engage them in more exciting and nuanced ways – to reveal fresh perspectives. AI empowers us to analyse a wider range of feedback (e.g. text, video, audio discussions, and open-ended responses).
For instance, our Whycatcher platform integrates with Whatsapp, allowing customers to provide real-time feedback in their preferred format – from text to video. We also leverage community panel platforms for discussion rooms and various feedback collection methods.
Open-ended responses from unstructured data can originate from a diverse array of sources (e.g. qualitative insights, audio content, discussion room interactions) and vary significantly from one customer to the next. Our bespoke AI toolkit tackles this challenge. It structures responses based on specific business questions, regardless of where the answers appear in the interview (beginning, middle, or end).
Additionally, we can layer on topic modelling and emotion detection to identify themes, their relative size or importance, and even interact with the data through strat7GPT. This approach unlocks deeper insights and reveals hidden patterns on a scale far exceeding traditional qualitative methods.
If there’s one takeaway, it’s this…
In our next and final blog in the series, we’ll debunk AI Myth #5: AI is limited in delivering customer centricity, and we explore how AI actually strengthens your customer connections.