Previously, we explored the power of human-AI collaboration in market research, highlighting how AI augments our capabilities rather than replacing them.
This time, we’re tackling a common concern, the perceived lack of representativeness in online data.
Generative AI isn’t representative, but AI can be
While generic generative AI tools might struggle with representativeness, bespoke and controlled AI can address this challenge. Here’s why:
A generic generative AI tool was tasked with understanding consumer television reviews. The response offered some inspiration but not at the level required to inform key decisions about the brand and its products. Whilst it gave a few examples of the conversation topics, it did not say how prominent those topics were. Further probing revealed the tool based its conclusions on the first five reviews it found – clearly, not representative at all!
However, as practitioners, we can control the sources. For example, we’re able to gather 100,000s of TV reviews in a couple of days. We can then filter those responses to be sure they are TV reviews (not based on fridges made by the same brands), and deep dive into the meta data surrounding the reviews (e.g. digging into smart TVs produced by a specified set of brands).
If we humans are controlling the sources, we remove the black box, and help frame the context, to deliver recommendations with more impact.
Context is key
Beyond controlling data sources, human understanding plays a crucial role in extracting the most value from online data. For instance, holiday reviews typically showcase both positive and negative experiences. However, review sites and social media for some categories might function primarily as complaint platforms. In these cases, our analytical approach will shift to prioritise identifying pain points and gauging the extent of customer dissatisfaction.
Another important point to make. Much of the online data on review sites, blogs and forums are in fact most representative of what consumers see in their online decision-making journey. Ignore this at your peril.
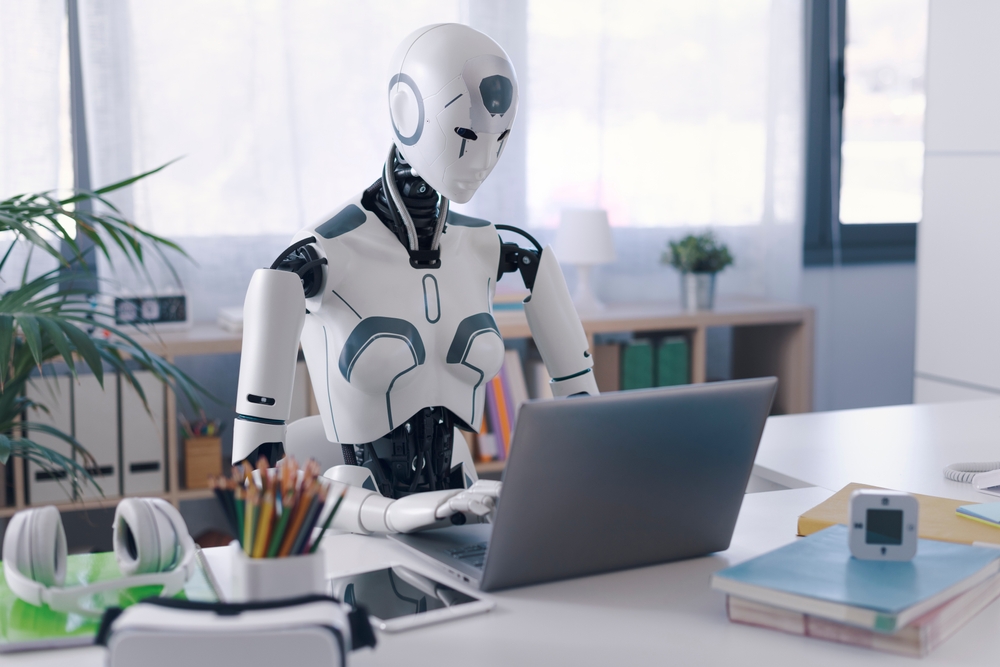
The power of combining AI and primary research
While online data on a small scale is not useful, collecting it on a massive scale can help to quantify brand performance.
We recently worked with a client who was looking to understand the European budget hotel market in more detail. By collecting data across many different hotel chains, we had enough sample to reliably analyse strengths and weaknesses by topic area and competitor. We were also able to segment the market into large chains and disrupters, clustering the conversation of the latter to identify themes driving their behaviour.
By combining these AI outputs with qual and quant, we achieved a comprehensive 360-degree view of the market. This holistic understanding empowered the client to develop a strategic positioning plan for achieving sustainable growth.
In summary…
In this post, we explored how bespoke AI, combined with human expertise, can overcome representativeness concerns by controlling data sources and understanding context. We also highlighted the power of combining AI with traditional research methods (qual and quant) for a comprehensive view of the market.
STRAT7 is methodology agnostic. We take a business question and then look to the sources, methods and expertise within STRAT7 to answer those questions in the best possible way. Grasping the context of the questions we’re addressing and recognising when to complement this with other data sources, ensures our recommendations are robust and reliable.
Check out the next blog in our series which covers AI Myth #4: AI can’t quantify challenges and opportunities at scale.