To properly test our new tool we compared the results from a human panel with our segment chatbot.
Welcome to our fourth blog on STRAT7’s new AI product – our segment chatbot.
To help show how a chatbot will transform an insight division (and beyond), here we outline a recent test case. We used STRAT7 Audience’s panel to survey 500 UK based adults on product ideas for new casual dining restaurants, and assigned the same task to a prototype chatbot trained on a self-funded segmentation study and its deliverables.
We compared a new restaurant idea written by a researcher well versed in the segmentation with a concept improved by the chatbot to appeal to a specific customer group. This improvement took the researcher’s concept and adjusted the messaging based on everything it knew about the group, from qual and quant data on the segments.
Here are the key findings in our comparison
Q1: Can the chatbot improve the quality of written comms – to therefore increase a chance of triggering a positive response?
Result: The chatbot version improved positive responses.
By analysing actual response rates in the survey, we could attribute the difference to the chatbot alone – i.e. a gold standard AB Test. (A = human researcher concept, B = AI optimised concept)
What did we find?
We found a significant improvement in the target customer group’s appeal in Concept B vs A. The target group were 7pp more likely to agree the concept was relevant to them, equivalent to a total attributable market increase of 670,000 UK adults.
Not only that, but it made the concept more differentiating. The target group was now 20pp more likely to find the concept appealing than any other group in B. This compared more favourably to A, where the target group was surprisingly behind other segment groups in its self-reported appeal.
Takeaway
The chatbot can genuinely help improve written comms and focus the innovation funnel to appeal to specific audiences.
Q2: Can the chatbot help to test early innovation ideas?
Result: The chatbot had higher accuracy rates of customer opinion.
We asked the chatbot to predict which customer group find each idea most exciting and then compared this to actual results in the panel.
What did we find?
We found the chatbot could predict the top two customer groups 90% of the time.
This compares to just 50% for an out of the box AI tool (like strat7GPT) – which only has access to the segment names and cannot reason using the underlying research outputs. And just 33% accuracy based on blind random guessing.
Takeaway
The chatbot is significantly better at making predictions against the ‘ground truth’ of a survey sample than other approaches.
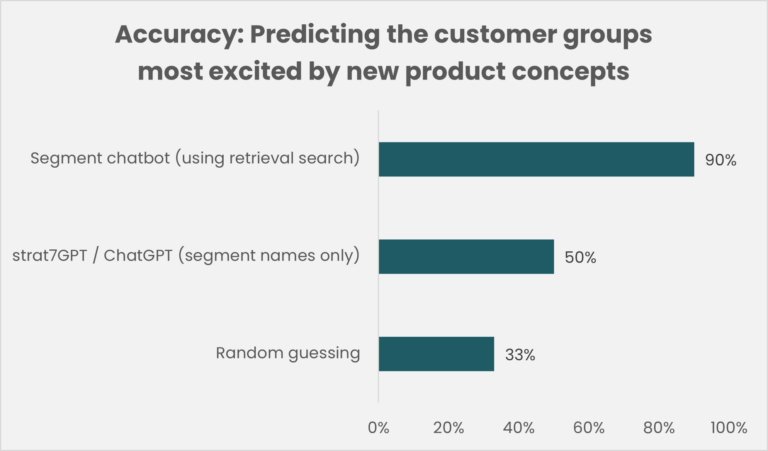
Conclusion
Ultimately, this test case shows how building a tool like STRAT7’s segment chatbot squeezes more out of existing research outputs with Generative AI and can genuinely save time, resources and boost ROI for insight teams.
Find out more
Contact us to find out more about how the STRAT7 segment chatbot can supercharge your research, or email me directly:
Hasdeep Sethi
Data Science Director & STRAT7 AI Lead
STRAT7 Bonamy Finch
hasdeep.sethi@bonamyfinch.com
Infographic
Segment chatbot
Want to talk to customers, as if they were in the room with you?
View our segment chatbot infographic to find out more, including what it is and what it can be used for.
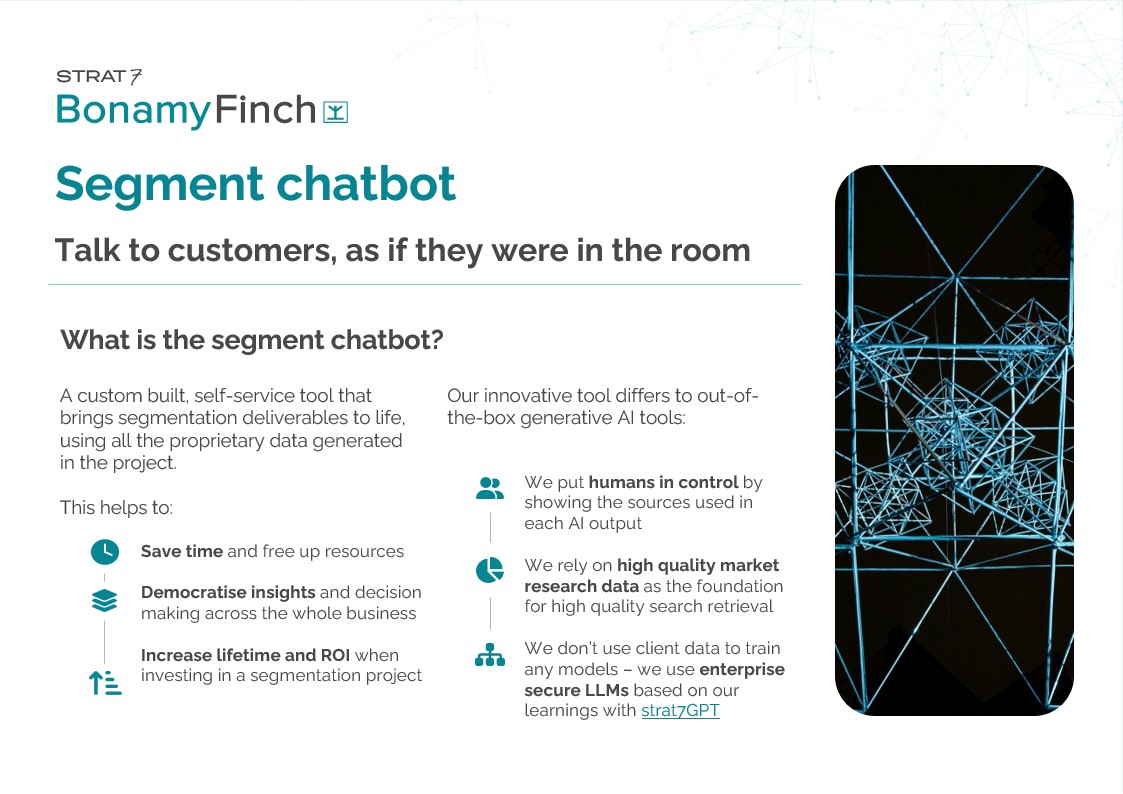