Artificial intelligence (AI) has received a huge amount of media interest in the past few years, and rightly so, it has increased capabilities in many areas. These models excel at deciphering and predicting complex patterns in data, whether identifying content in images or forecasting future sales, and can outperform standard models in various tasks.
However, this power comes with a caveat – these models need vast amounts of data and significant computational power to be trained. Contrary to the AI hype, complex modelling isn’t always the best solution.
In this article, we advocate for the benefits of simplicity.
The power of simplicity
Simpler models like regression, decision trees, and support vector machines can be more effective for predictive tasks. These models offer several key benefits:
1. Ease of interpretation
One of the significant advantages of simpler models is their transparency. Stakeholders can easily understand how these models work and how decisions are made. This transparency fosters trust and ensures that insights derived from the models are more likely to be acted upon.
2. Incorporating domain expertise
Simple models allow you to embed domain knowledge directly into the model. For instance, you can set constraints on variables to ensure they align with real-world expectations. This built-in expertise acts as a safeguard, ensuring the model’s outputs are practical, relevant and that insights derived from the models are more likely to be acted upon.
3. Better generalisation
When data is limited, simpler models are often better at generalising from the available information. They do not fit the data too tightly and are less likely to capture noise as meaningful patterns. In contrast, complex models can overfit, incorporating biases and noise if not carefully controlled. Simpler models therefore, tend to perform more reliably on unseen data.
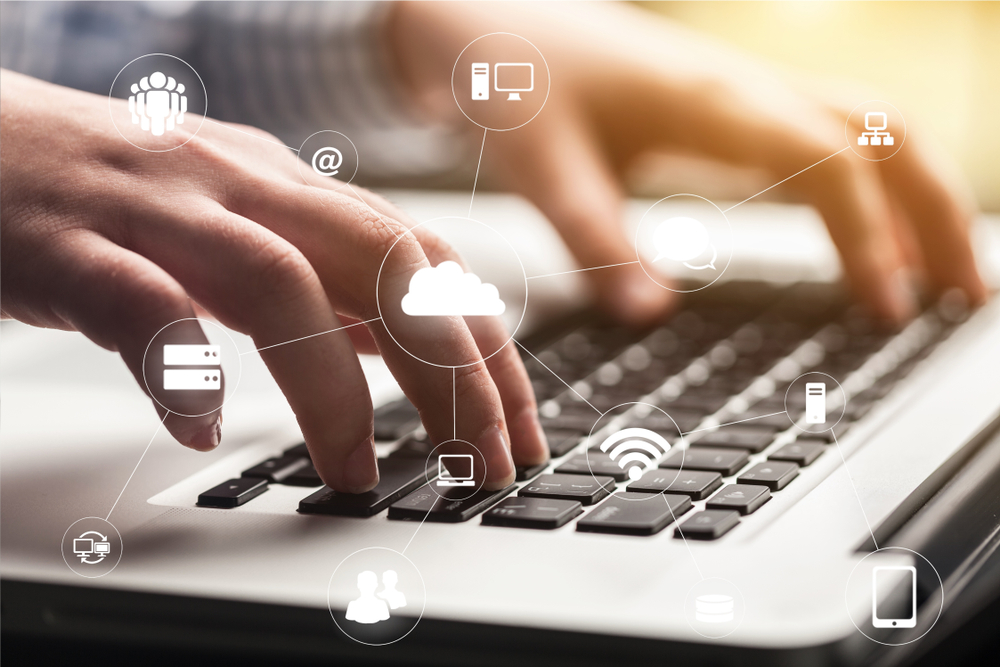
Additional considerations
1. Scalability
Traditional models are often easier and less costly to scale compared to complex AI models. Businesses with limited resources can implement and maintain these models without the need for extensive infrastructure or computational power.
2. Speed of implementation
Simpler models typically require less time to develop and deploy. This can be crucial for businesses needing quick insights to make timely decisions.
3. Cost-effectiveness
Without the need for vast datasets and high computational power, traditional models can be more cost-effective. This is particularly beneficial for small to medium-sized enterprises operating on tighter budgets.
Practical implementation
In real-world applications, the practical implementation of predictive models is often just as important as their theoretical capabilities. Simpler models can be quickly adapted to changing business needs and can be more easily integrated into existing systems.
This adaptability ensures that businesses can remain agile and responsive to new information, making timely adjustments without the need for extensive retraining or computational overhead. This practical approach can be especially valuable in fast-paced industries where quick decision-making is crucial.
Conclusion
When we think of predictive modelling work, it’s crucial to balance the allure of complexity with the practical benefits of simplicity. By leveraging human expertise and ensuring transparency, traditional models not only deliver accurate predictions but also build trust and comply with regulatory standards. Embracing simplicity can lead to better results, especially when resources and data are limited.
If you’re interested in finding out more please don’t hesitate to get in touch, or contact Steven Pesarra directly at steven.pesarra@bonamyfinch.com.
Ebook
Unlock the commercial value of your data
Download our ebook to learn how to create an effective data-driven culture by integrating high-quality data into everything you do – helping you become more customer-centric, make better decisions, and surpass your revenue goals.