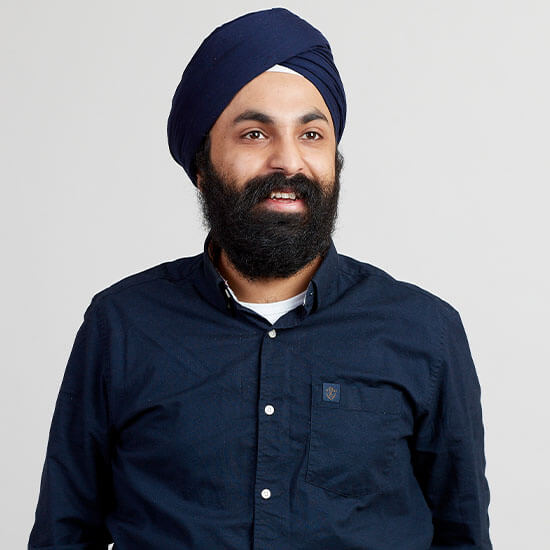
Hasdeep Sethi, Data Science Director at Bonamy Finch and strat7.ai Data Science Lead, is well placed to predict what’s coming up in the world of AI. He navigates us through the evolving landscape with his top five predictions for the year ahead.
To those reading this, it will be of no surprise that ‘AI’ was named as the Collins word (or should that be acronym?) of the year in 2023.
And now, 15 months after the first release of Open AI’s ChatGPT and many competitors catching up, 2024 will surely be a year where AI moves from ‘experimentation’ and ‘understanding’ to ‘production’ – helping to save lots of time and unlock new insights in market research.
While there will be a flurry of AI developments this year, here are my top five (almost guaranteed) predictions for the year ahead:
1. Large Language Models (LLMs) go multimodal
Models like GPT-4 and Google Gemini now offer breakthrough capabilities that equip models with ‘see’, ‘hear’, and ‘speak’ capabilities, beyond just being able to ‘read’ text. This means models are moving from a world of ‘text in, text out’ to a rich set of inputs and outputs.
Therefore, it’s now possible to combine multiple unstructured sources (e.g. upload a video and get a summary back, or provide a written description and get a set of curated images back) with enterprise grade models.
This is important, as people often prefer visual stimuli to just written outputs. And LLMs are now able to do this.
2. Pre-processing the data that feed LLMs
Our development of strat7GPT has taught us that enterprise grade LLMs are very good at summarising text in an easy to read format (e.g. transcripts, survey open ends, PDF documents) out of the box.
However, summarising media like PowerPoint charts and Excel tables is much more challenging and can easily trigger hallucination in LLM responses.
This requires tailored processing rules to teach the models ‘where to look’. While open-source libraries help, new market entrants will help to simplify the process of ‘talking to’ complex data types in the coming months.
3. Giving LLMs more ‘long-term’ memory
While using strat7GPT to help build tools for our clients, we have found that RAG search sometimes isn’t quite enough to make LLMs sufficiently ‘intelligent’. Even with clear prompt engineering guidelines for users and rigorous data pre-processing in the backend, ‘out of the box’ LLMs are sometimes not personalised enough – especially when it comes to understanding market research jargon.
To improve the user experience, it’s necessary to fine tune LLMs with a set of instructions, such that it acquires ‘long-term memory’. This could be a glossary of terms that is widely understood in a company or some clear Q&A examples. This transforms LLMs from a general purpose assistant into a domain specific assistant.
Adoption of fine tuning will therefore surely accelerate in the months ahead.
4. LLMs replacing and competing with traditional machine learning tasks
In traditional machine learning, algorithms rely on historical data to establish patterns which are then applied to unseen data to make predictions. LLMs reduce this barrier to entry by removing the requirement of historical data.
For us at STRAT7, that has meant creatively prompting LLMs to extract useful information from text, like location or company name – instead of relying on pre-trained algorithms.
We’ve also started to integrate it with our existing toolkit to help improve the accuracy of our in-house topic modelling algorithms.
5. Opening up of new research outputs and tools
Last and by no means least, is the opening up of entirely new research outputs, which is something we’ve been working on at STRAT7.
As people become more familiar with AI in research, tools like the below are moving into the spotlight:
Segmentation chatbots: Being able to talk to segments to trial concepts and extract information can help with the adoption of segmentations in a large business.
Competitor intelligence tools: Ingesting and storing lots of publicly available unstructured data on competitors (e.g. social media, forums, blogs, reviews, news sites) and asking questions to an LLM can help to democratise competitor intelligence. While not a replacement for traditional desk research and survey-based brand trackers, it is something that our clients are now seriously thinking about.
Searchable qual interview databases: Interviewing dozens or even 100s of people with auto transcription and categorisation enabled in a searchable database will enable ‘thick’ qual, which combines the richness of qual research with the rigour of quant research.
There are many opportunities in market research to embrace the developments in AI.
If you feel you’re not taking full advantage of what’s on offer or how to make this work for you’re business just get in touch.